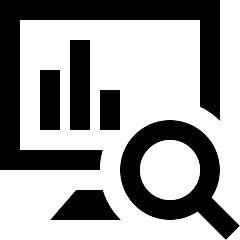
Data Handling with R and SQL
preface 이번 포스트에서는 R 과 SQL 을 이용하여 데이터프레임을 조작하는 방법을 다룹니다. 많이 쓰이는 대표적인 경우를 R 과 SQL 각각 어떻게 코딩하는지 살펴봅니다.
다음 자료를 참고하였습니다:
- https://cran.r-project.org/web/packages/sqldf/index.html
- 위 링크에 있는 Reference manual 의 예제를 변형하였습니다.
sqldf 패키지 설치
일반적으로 R 에서 패키지 설치는 R studio 에서 packages > install 통해 가능합니다. 하지만 jupyter notebook 을 통해 R 을 사용하는 경우에는 다음을 입력합시다.
install.packages('sqldf', repos='http://cran.us.r-project.org')
샘플 데이터 생성
대부분의 경우 IRIS 데이터를 샘플로 사용합니다. sqldf
를 사용하면 db를 따로 만드는 번거로움을 생략할 수 있지만, 어쩐일인지 요즘 제 컴퓨터에서 sqldf
가 에러가 나는 관계로 RSQLite
를 사용하겠습니다.
# These examples show how to run a variety of data frame manipulations
# in R without SQL and then again with SQL
library(sqldf)
library(RSQLite)
dfIRIS <- iris
fctr.cols <- sapply(dfIRIS, is.factor)
dfIRIS[, fctr.cols] <- sapply(dfIRIS[, fctr.cols], as.character)
names(dfIRIS)[1] = 'Sepal_Length'
names(dfIRIS)[2] = 'Sepal_Width'
names(dfIRIS)[3] = 'Petal_Length'
names(dfIRIS)[4] = 'Petal_Width'
conn <- dbConnect(SQLite(),'mydb.db')
dbWriteTable(conn, "dfIRIS", dfIRIS, overwrite = TRUE)
Subset
head
# head - 처음 n 개 obs 추출
a1r <- head(dfIRIS, n=5)
a1s <- dbGetQuery(conn, "select * from dfIRIS limit 5")
identical(a1r, a1s)
TRUE
a1r
Sepal_Length | Sepal_Width | Petal_Length | Petal_Width | Species |
---|---|---|---|---|
5.1 | 3.5 | 1.4 | 0.2 | setosa |
4.9 | 3.0 | 1.4 | 0.2 | setosa |
4.7 | 3.2 | 1.3 | 0.2 | setosa |
4.6 | 3.1 | 1.5 | 0.2 | setosa |
5.0 | 3.6 | 1.4 | 0.2 | setosa |
조건: 특정 문자열 포함
# subset - feature 가 특정 문자열을 포함한 obs 추출
a2r <- subset(dfIRIS, grepl("^se", Species))
a2s <- dbGetQuery(conn, "select * from dfIRIS where Species like 'se%'")
all.equal(as.data.frame(a2r), a2s)
TRUE
head(a2r, n=5)
Sepal_Length | Sepal_Width | Petal_Length | Petal_Width | Species |
---|---|---|---|---|
5.1 | 3.5 | 1.4 | 0.2 | setosa |
4.9 | 3.0 | 1.4 | 0.2 | setosa |
4.7 | 3.2 | 1.3 | 0.2 | setosa |
4.6 | 3.1 | 1.5 | 0.2 | setosa |
5.0 | 3.6 | 1.4 | 0.2 | setosa |
조건: 특정 categories 포함
#subset - feature 가 특정 이름(들)을 가진 obs 추출
a3r <- subset(dfIRIS, Species %in% c("setosa", "versicolor"))
a3s <- dbGetQuery(conn, "select * from dfIRIS where Species in ('setosa', 'versicolor')")
row.names(a3r) <- NULL
identical(a3r, a3s)
TRUE
head(a3r, n=5)
Sepal_Length | Sepal_Width | Petal_Length | Petal_Width | Species |
---|---|---|---|---|
5.1 | 3.5 | 1.4 | 0.2 | setosa |
4.9 | 3.0 | 1.4 | 0.2 | setosa |
4.7 | 3.2 | 1.3 | 0.2 | setosa |
4.6 | 3.1 | 1.5 | 0.2 | setosa |
5.0 | 3.6 | 1.4 | 0.2 | setosa |
조건: 특정 변수의 범위 지정
# subset - feature 가 특정 범위 안에 있는 obs 추출
a4r <- subset(dfIRIS, Sepal_Length >= 5.5 & Sepal_Length <= 5.6)
a4s <- dbGetQuery(conn, "select * from dfIRIS where Sepal_Length between 5.5 and 5.6")
row.names(a4r) <- NULL
identical(a4r, a4s)
TRUE
a4r
Sepal_Length | Sepal_Width | Petal_Length | Petal_Width | Species |
---|---|---|---|---|
5.5 | 4.2 | 1.4 | 0.2 | setosa |
5.5 | 3.5 | 1.3 | 0.2 | setosa |
5.5 | 2.3 | 4.0 | 1.3 | versicolor |
5.6 | 2.9 | 3.6 | 1.3 | versicolor |
5.6 | 3.0 | 4.5 | 1.5 | versicolor |
5.6 | 2.5 | 3.9 | 1.1 | versicolor |
5.5 | 2.4 | 3.8 | 1.1 | versicolor |
5.5 | 2.4 | 3.7 | 1.0 | versicolor |
5.6 | 3.0 | 4.1 | 1.3 | versicolor |
5.5 | 2.5 | 4.0 | 1.3 | versicolor |
5.5 | 2.6 | 4.4 | 1.2 | versicolor |
5.6 | 2.7 | 4.2 | 1.3 | versicolor |
5.6 | 2.8 | 4.9 | 2.0 | virginica |
조건: 특정 값
# subset - feature 가 특정 값을 가진 obs 추출
a5r <- subset(dfIRIS, Species == 'versicolor')
a5s <- dbGetQuery(conn, "select * from dfIRIS where Species = 'versicolor'")
row.names(a5r) <- NULL
identical(a5r, a5s)
TRUE
head(a5r, n=5)
Sepal_Length | Sepal_Width | Petal_Length | Petal_Width | Species |
---|---|---|---|---|
7.0 | 3.2 | 4.7 | 1.4 | versicolor |
6.4 | 3.2 | 4.5 | 1.5 | versicolor |
6.9 | 3.1 | 4.9 | 1.5 | versicolor |
5.5 | 2.3 | 4.0 | 1.3 | versicolor |
6.5 | 2.8 | 4.6 | 1.5 | versicolor |
a6r <- subset(dfIRIS, Species == 'virginica')
a6s <- dbGetQuery(conn, "select * from dfIRIS where Species = 'virginica'")
row.names(a6r) <- NULL
identical(a6r, a6s)
TRUE
head(a6r, n=5)
Sepal_Length | Sepal_Width | Petal_Length | Petal_Width | Species |
---|---|---|---|---|
6.3 | 3.3 | 6.0 | 2.5 | virginica |
5.8 | 2.7 | 5.1 | 1.9 | virginica |
7.1 | 3.0 | 5.9 | 2.1 | virginica |
6.3 | 2.9 | 5.6 | 1.8 | virginica |
6.5 | 3.0 | 5.8 | 2.2 | virginica |
rbind
# rbind - 동일한 features 를 가진 두 데이터를 위아래로 연결
a7r <- rbind(a5r, a6r)
dbWriteTable(conn, "a5s", a5s, overwrite = TRUE)
dbWriteTable(conn, "a6s", a6s, overwrite = TRUE)
a7s <- dbGetQuery(conn, "select * from a5s union all select * from a6s")
identical(a7r, a7s)
TRUE
자료의 기술통계량 (평균 등)
# aggregate - 그룹별 평균: 각 Species 별 Sepal_Length 와 Sepal_Width 의 평균을 계산
# aggregate - avg conc and uptake by Plant and Type
a8r <- aggregate(dfIRIS[1:2], dfIRIS[5], mean)
a8s <- dbGetQuery(conn, 'select Species, avg("Sepal_Length") `Sepal_Length`,
avg("Sepal_Width") `Sepal_Width` from dfIRIS group by Species')
all.equal(a8r, a8s)
TRUE
a8r
Species | Sepal_Length | Sepal_Width |
---|---|---|
setosa | 5.006 | 3.428 |
versicolor | 5.936 | 2.770 |
virginica | 6.588 | 2.974 |
# by - 각 Species 별 Sepal_Length, Sepal_Width, Sepal_ratio 의 평균을 계산
# by - avg conc and total uptake by Plant and Type
a9r <- do.call(rbind, by(dfIRIS, dfIRIS[5], function(x) with(x,
data.frame(Species = Species[1],
mean.Sepal_Length = mean(Sepal_Length),
mean.Sepal_Width = mean(Sepal_Width),
mean.Sepal_ratio = mean(Sepal_Length/Sepal_Width)))))
row.names(a9r) <- NULL
a9s <- dbGetQuery(conn, 'select Species, avg("Sepal_Length") `mean.Sepal_Length`,
avg("Sepal_Width") `mean.Sepal_Width`,
avg("Sepal_Length"/"Sepal_Width") `mean.Sepal_ratio` from dfIRIS
group by Species')
fctr.cols <- sapply(a9r, is.factor)
a9r[, fctr.cols] <- sapply(a9r[, fctr.cols], as.character)
all.equal(a9r, a9s)
TRUE
a9r
Species | mean.Sepal_Length | mean.Sepal_Width | mean.Sepal_ratio |
---|---|---|---|
setosa | 5.006 | 3.428 | 1.470188 |
versicolor | 5.936 | 2.770 | 2.160402 |
virginica | 6.588 | 2.974 | 2.230453 |
Sort
# order - 특정 변수(Sepal_Length)의 크기순으로 정렬하고, 가장 큰 3개 obs 추출
a10r <- head(dfIRIS[order(dfIRIS$Sepal_Length, decreasing = TRUE), ], 3)
a10s <- dbGetQuery(conn, "select * from dfIRIS order by Sepal_Length desc limit 3")
row.names(a10r) <- NULL
identical(a10r, a10s)
TRUE
a10s
Sepal_Length|Sepal_Width|Petal_Length|Petal_Width|Species ————|———–|————|———–|——– 7.9 | 3.8 | 6.4 | 2.0 | virginica 7.7 | 3.8 | 6.7 | 2.2 | virginica 7.7 | 2.6 | 6.9 | 2.3 | virginica
# order - 특정 변수(Sepal_Length)의 크기순으로 정렬하고, 가장 작은 3개 obs 추출
a11r <- head(dfIRIS[order(dfIRIS$Sepal_Length), ], 3)
a11s <- dbGetQuery(conn, "select * from dfIRIS order by Sepal_Length limit 3")
# attributes(a11r) <- attributes(a11s) <- NULL
row.names(a11r) <- NULL
identical(a11r, a11s)
TRUE
a11s
Sepal_Length | Sepal_Width | Petal_Length | Petal_Width | Species |
---|---|---|---|---|
4.3 | 3.0 | 1.1 | 0.1 | setosa |
4.4 | 2.9 | 1.4 | 0.2 | setosa |
4.4 | 3.0 | 1.3 | 0.2 | setosa |
# order
a17r <- dfIRIS[order(dfIRIS$Sepal_Length, decreasing = TRUE), ]
a17s <- dbGetQuery(conn, "select * from dfIRIS order by Sepal_Length desc")
row.names(a17r) <- NULL
identical(a17r, a17s)
TRUE
head(a17r, n=5)
Sepal_Length | Sepal_Width | Petal_Length | Petal_Width | Species |
---|---|---|---|---|
7.9 | 3.8 | 6.4 | 2.0 | virginica |
7.7 | 3.8 | 6.7 | 2.2 | virginica |
7.7 | 2.6 | 6.9 | 2.3 | virginica |
7.7 | 2.8 | 6.7 | 2.0 | virginica |
7.7 | 3.0 | 6.1 | 2.3 | virginica |
# ave - 그룹별 평균을 초과하는 관측치 추출
# ave - rows for which v exceeds its group average where g is group
DF <- data.frame(g = rep(1:2, each = 5), t = rep(1:5, 2), v = 1:10)
a12r <- subset(DF, v > ave(v, g, FUN = mean))
dbWriteTable(conn, "DF", DF, overwrite = TRUE)
Gavg <- dbGetQuery(conn, "select g, avg(v) as avg_v from DF group by g")
dbWriteTable(conn, "Gavg", Gavg, overwrite = TRUE)
a12s <- dbGetQuery(conn, "select DF.g, t, v from DF, Gavg where DF.g = Gavg.g and v > avg_v")
row.names(a12r) <- NULL
identical(a12r, a12s)
TRUE
DF
g | t | v |
---|---|---|
1 | 1 | 1 |
1 | 2 | 2 |
1 | 3 | 3 |
1 | 4 | 4 |
1 | 5 | 5 |
2 | 1 | 6 |
2 | 2 | 7 |
2 | 3 | 8 |
2 | 4 | 9 |
2 | 5 | 10 |
a12r
g | t | v |
---|---|---|
1 | 4 | 4 |
1 | 5 | 5 |
2 | 4 | 9 |
2 | 5 | 10 |
# same but reduce the two select statements to one using a subquery
a13s <- dbGetQuery(conn, "select g, t, v
from DF d1, (select g as g2, avg(v) as avg_v from DF group by g)
where d1.g = g2 and v > avg_v")
identical(a12r, a13s)
TRUE
# same but shorten using natural join
a14s <- dbGetQuery(conn, "select g, t, v
from DF
natural join (select g, avg(v) as avg_v from DF group by g)
where v > avg_v")
identical(a12r, a14s)
TRUE
Frequency Table by Group
# table
a15r <- table(warpbreaks$tension, warpbreaks$wool)
dbWriteTable(conn, "warpbreaks", warpbreaks, overwrite = TRUE)
a15s <- dbGetQuery(conn, "select sum(wool = 'A'), sum(wool = 'B')
from warpbreaks group by tension")
all.equal(as.data.frame.matrix(a15r), a15s, check.attributes = FALSE)
TRUE
a15r
0 | A | B |
---|---|---|
L | 9 | 9 |
H | 9 | 9 |
M | 9 | 9 |
# reshape - 그룹(g) x 그룹(t)의 관측치(v)
t.names <- paste("t", unique(as.character(DF$t)), sep = "_")
a16r <- reshape(DF, direction = "wide", timevar = "t", idvar = "g", varying = list(t.names))
a16s <- dbGetQuery(conn, "select
g,
sum((t == 1) * v) t_1,
sum((t == 2) * v) t_2,
sum((t == 3) * v) t_3,
sum((t == 4) * v) t_4,
sum((t == 5) * v) t_5
from DF group by g")
all.equal(a16r, a16s, check.attributes = FALSE)
a16s
g | t_1 | t_2 | t_3 | t_4 | t_5 |
---|---|---|---|---|---|
1 | 1 | 2 | 3 | 4 | 5 |
2 | 6 | 7 | 8 | 9 | 10 |
Moving Average
# centered moving average of length 7 - 이동평균
set.seed(1)
DF <- data.frame(x = rnorm(15, 1:15))
DF$row_names <- c(1:15)
DF
x | row_names |
---|---|
0.3735462 | 1 |
2.1836433 | 2 |
2.1643714 | 3 |
5.5952808 | 4 |
5.3295078 | 5 |
5.1795316 | 6 |
7.4874291 | 7 |
8.7383247 | 8 |
9.5757814 | 9 |
9.6946116 | 10 |
12.5117812 | 11 |
12.3898432 | 12 |
12.3787594 | 13 |
11.7853001 | 14 |
16.1249309 | 15 |
dbWriteTable(conn, "DF", DF, overwrite = TRUE)
a18s <- dbGetQuery(conn, "select a.x 'x.x', a.row_names 'x.row_names', avg(b.x) movavgx from DF a, DF b
where a.row_names - b.row_names between -3 and 3
group by a.row_names having count(*) = 7
order by a.row_names+0",
row.names = FALSE)
a18r <- data.frame(x = DF[4:12,], movavgx = rowMeans(embed(DF$x, 7)))
row.names(a18r) <- NULL
all.equal(a18r, a18s)
TRUE
a18r
x.x | x.row_names | movavgx |
---|---|---|
5.595281 | 4 | 4.044759 |
5.329508 | 5 | 5.239727 |
5.179532 | 6 | 6.295747 |
7.487429 | 7 | 7.371495 |
8.738325 | 8 | 8.359567 |
9.575781 | 9 | 9.368186 |
9.694612 | 10 | 10.396647 |
12.511781 | 11 | 11.010629 |
12.389843 | 12 | 12.065858 |
Merge
# merge. a19r and a19s are same except row order and row names
A <- data.frame(a1 = c(1, 2, 1), a2 = c(2, 3, 3), a3 = c(3, 1, 2))
B <- data.frame(b1 = 1:2, b2 = 2:1)
A
B
a1 | a2 | a3 |
---|---|---|
1 | 2 | 3 |
2 | 3 | 1 |
1 | 3 | 2 |
b1 | b2 |
---|---|
1 | 2 |
2 | 1 |
a19r <- merge(A, B)
a19r
dbWriteTable(conn, "A", A, overwrite = TRUE)
dbWriteTable(conn, "B", B, overwrite = TRUE)
a19s <- dbGetQuery(conn, "select * from A, B")
a19s
Sort <- function(DF) DF[do.call(order, DF),]
all.equal(Sort(a19s), Sort(a19r), check.attributes = FALSE)
a1 | a2 | a3 | b1 | b2 |
---|---|---|---|---|
1 | 2 | 3 | 1 | 2 |
2 | 3 | 1 | 1 | 2 |
1 | 3 | 2 | 1 | 2 |
1 | 2 | 3 | 2 | 1 |
2 | 3 | 1 | 2 | 1 |
1 | 3 | 2 | 2 | 1 |
a1 | a2 | a3 | b1 | b2 |
---|---|---|---|---|
1 | 2 | 3 | 1 | 2 |
1 | 2 | 3 | 2 | 1 |
2 | 3 | 1 | 1 | 2 |
2 | 3 | 1 | 2 | 1 |
1 | 3 | 2 | 1 | 2 |
1 | 3 | 2 | 2 | 1 |
# within Date, of the highest quality records list the one closest
# to noon. Note use of two sql statements in one call to sqldf.
Lines <- "DeployID Date.Time LocationQuality Latitude Longitude
STM05-1 2005/02/28 17:35 Good -35.562 177.158
STM05-1 2005/02/28 19:44 Good -35.487 177.129
STM05-1 2005/02/28 23:01 Unknown -35.399 177.064
STM05-1 2005/03/01 07:28 Unknown -34.978 177.268
STM05-1 2005/03/01 18:06 Poor -34.799 177.027
STM05-1 2005/03/01 18:47 Poor -34.85 177.059
STM05-2 2005/02/28 12:49 Good -35.928 177.328
STM05-2 2005/02/28 21:23 Poor -35.926 177.314
"
DF <- read.table(textConnection(Lines), skip = 1, as.is = TRUE,
col.names = c("Id", "Date", "Time", "Quality", "Lat", "Long"))
DF
Id | Date | Time | Quality | Lat | Long |
---|---|---|---|---|---|
STM05-1 | 2005/02/28 | 17:35 | Good | -35.562 | 177.158 |
STM05-1 | 2005/02/28 | 19:44 | Good | -35.487 | 177.129 |
STM05-1 | 2005/02/28 | 23:01 | Unknown | -35.399 | 177.064 |
STM05-1 | 2005/03/01 | 07:28 | Unknown | -34.978 | 177.268 |
STM05-1 | 2005/03/01 | 18:06 | Poor | -34.799 | 177.027 |
STM05-1 | 2005/03/01 | 18:47 | Poor | -34.850 | 177.059 |
STM05-2 | 2005/02/28 | 12:49 | Good | -35.928 | 177.328 |
STM05-2 | 2005/02/28 | 21:23 | Poor | -35.926 | 177.314 |
dbWriteTable(conn, "DF", DF, overwrite = TRUE)
DF1 <- dbGetQuery(conn, "select * from DF
order by Date DESC, Quality DESC, abs(substr(Time, 1, 2) + substr(Time, 4, 2) /60 - 12) DESC")
dbWriteTable(conn, "DF1", DF1, overwrite = TRUE)
DF1
a20s <- dbGetQuery(conn, "select * from DF1 group by Date")
a20s
# sqldf(c("create temp table DF1 as select * from DF order by
# Date DESC, Quality DESC,
# abs(substr(Time, 1, 2) + substr(Time, 4, 2) /60 - 12) DESC",
# "select * from DFo group by Date"))
TRUE
Id | Date | Time | Quality | Lat | Long |
---|---|---|---|---|---|
STM05-1 | 2005/03/01 | 07:28 | Unknown | -34.978 | 177.268 |
STM05-1 | 2005/03/01 | 18:06 | Poor | -34.799 | 177.027 |
STM05-1 | 2005/03/01 | 18:47 | Poor | -34.850 | 177.059 |
STM05-1 | 2005/02/28 | 23:01 | Unknown | -35.399 | 177.064 |
STM05-2 | 2005/02/28 | 21:23 | Poor | -35.926 | 177.314 |
STM05-1 | 2005/02/28 | 19:44 | Good | -35.487 | 177.129 |
STM05-1 | 2005/02/28 | 17:35 | Good | -35.562 | 177.158 |
STM05-2 | 2005/02/28 | 12:49 | Good | -35.928 | 177.328 |
Id | Date | Time | Quality | Lat | Long |
---|---|---|---|---|---|
STM05-2 | 2005/02/28 | 12:49 | Good | -35.928 | 177.328 |
STM05-1 | 2005/03/01 | 18:47 | Poor | -34.850 | 177.059 |
#Outer join:
merge(x = df1, y = df2, by = "CustomerId", all = TRUE)
#Left outer:
merge(x = df1, y = df2, by = "CustomerId", all.x = TRUE)
#Right outer:
merge(x = df1, y = df2, by = "CustomerId", all.y = TRUE)
#Cross join:
merge(x = df1, y = df2, by = NULL)
etc
## Not run:
# test of file connections with sqldf
# create test .csv file of just 3 records
write.table(head(dfIRIS, 3), "dfIRIS3.dat", sep = ",", quote = FALSE)
# look at contents of dfIRIS3.dat
readLines("dfIRIS3.dat")
# set up file connection
dfIRIS3 <- file("dfIRIS3.dat")
sqldf('select * from dfIRIS3 where "Sepal_Width" > 3')
# using a non-default separator
# file.format can be an attribute of file object or an arg passed to sqldf
write.table(head(dfIRIS, 3), "dfIRIS3.dat", sep = ";", quote = FALSE)
dfIRIS3 <- file("dfIRIS3.dat")
sqldf('select * from dfIRIS3 where "Sepal_Width" > 3', file.format = list(sep = ";"))
# same but pass file.format through attribute of file object
attr(dfIRIS3, "file.format") <- list(sep = ";")
sqldf('select * from dfIRIS3 where "Sepal_Width" > 3')
# copy file straight to disk without going through R
# and then retrieve portion into R
sqldf('select * from dfIRIS3 where "Sepal_Width" > 3', dbname = tempfile())
### same as previous example except it allows multiple queries against
### the database. We use dfIRIS3 from before. This time we use an
### in memory SQLite database.
sqldf() # open a connection
sqldf('select * from dfIRIS3 where "Sepal_Width" > 3')
# At this point we have an dfIRIS3 variable in both
# the R workspace and in the SQLite database so we need to
# explicitly let it know we want the version in the database.
# If we were not to do that it would try to use the R version
# by default and fail since sqldf would prevent it from
# overwriting the version already in the database to protect
# the user from inadvertent errors.
sqldf('select * from main.dfIRIS3 where "Sepal_Width" > 4')
sqldf('select * from main.dfIRIS3 where "Sepal_Width" < 4')
sqldf() # close connection
### another way to do this is a mix of sqldf and RSQLite statements
### In that case we need to fetch the connection for use with RSQLite
### and do not have to specifically refer to main since RSQLite can
### only access the database.
con <- sqldf()
# this dfIRIS3 refers to the R variable and file
sqldf('select * from dfIRIS3 where "Sepal_Width" > 3')
sqldf("select count(*) from dfIRIS3")
# these dfIRIS3 refer to the database table
dbGetQuery(con, 'select * from dfIRIS3 where "Sepal_Width" > 4')
dbGetQuery(con, 'select * from dfIRIS3 where "Sepal_Width" < 4')
sqldf()
## End(Not run)