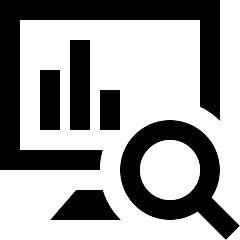
Logistic Regression
preface Logistic regression 은 종속변수가 0/1 로 구성된 binary outcome 이고 설명변수가 continuous 로 구성되어 있을 때 사용 가능한 분석 방법입니다. 모형 적용에 필요한 가정이 적기 때문에 discriminant analysis 보다 널리 사용됩니다.
다음 자료를 참고하였습니다:
Logistic, Logit, odds, odds ratio
$\text{logit function}$ :
.
.
$\text{logistic function}$ :
.
.
$\pi = F\left(X\right) = P \left( Y_i = 1 | X \right)$ : the probability of the event given $X$
$\text{odds}$ : the probability of the event divided by the probability of the event not occurring.
$\text{odds ratio}$ :
Model Fitting
Log Likelihood
.
Logistic Regression in R using glm()
# Logistic Regression
# where F is a binary factor and
# x1-x3 are continuous predictors
fit <- glm(F~x1+x2+x3,data=mydata,family=binomial())
summary(fit) # display results
confint(fit) # 95% CI for the coefficients
exp(coef(fit)) # exponentiated coefficients
exp(confint(fit)) # 95% CI for exponentiated coefficients
predict(fit, type="response") # predicted values
residuals(fit, type="deviance") # residuals
anova(fit1,fit2, test="Chisq")
함수를 사용하여 nested model 비교를 할 수 있습니다. cdplot(F~x, data=mydata)
함수를 사용하면 conditional density plot 을 그릴 수 있습니다.