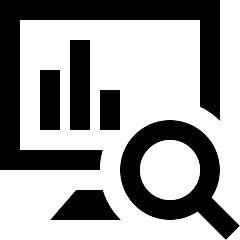
파이썬 기초 04 numpy and array
preface 이번 포스트에서는 파이썬을 이용하여 데이터분석을 할 수 있게 해주는 numpy 패키지에 대해 알아보겠습니다. numpy 패키지는 행렬 연산에 특화되어있어 matlab 과 유사하다는 인상을 받았습니다.
참고: Python 에서 중요한 라이브러리
- numpy
- pandas
- matplotlib
- scipy
- seaborn : 그래프가 웹브라우저에서 표현되도록 합니다.
행렬의 입력: array, matrix
import numpy as np
x=np.arange(10)
x # array([0, 1, 2, 3, 4, 5, 6, 7, 8, 9])
np.ones(10) # array([ 1., 1., 1., 1., 1., 1., 1., 1., 1., 1.])
# 리스트를 array로
income = [50000, 50000, 100000, 25000, 32100, 30000]
income # [50000, 50000, 100000, 25000, 32100, 30000]
income2 = np.array(income)
income2 # array([ 50000, 50000, 100000, 25000, 32100, 30000])
income2[0] # 50000
income2[2:] # array([100000, 25000, 32100, 30000])
income2[:2] # array([50000, 50000])
income2[[2,3,5]] # array([100000, 25000, 30000])
# matrix 를 사용할 수도 있습니다
np.matrix([[1,2],[3,4]])
행렬의 연산
# 행렬 matrix 연산
data1 = [1,2,3,4]
data2 = [5,6,7,8]
A = np.array(data1).reshape(2,2)
B = np.array(data2).reshape(2,2)
A # array([[1, 2],[3, 4]])
B # array([[5, 6],[7, 8]])
A+B # array([[ 6, 8],[10, 12]])
A-B # array([[-4, -4],[-4, -4]])
A*B # array([[ 5, 12],[21, 32]]) 행렬 곱이 아니라 같은 위치의 원소들끼리의 곱
A/B # array([[ 0.2 , 0.33333333],[ 0.42857143, 0.5 ]])
np.dot(A,B) # array([[19, 22],[43, 50]]) 행렬 곱
np.dot(B,A) # array([[23, 34],[31, 46]]) 행렬 곱
# numpy array의 주요 속성: shape, dtype, ndi,
A.ndim # 2
A.shape # (2, 2)
A.dtype # dtype('int32')
A.T # array([[1, 3],[2, 4]]) 전치행렬 transpose
np.diag(A) # array([1, 4]) 대각행렬 diagonal matrix
np.linalg.inv(A) # array([[-2. , 1. ],[ 1.5, -0.5]]) 역행렬 inverse matrix
np.linalg.eig(A) # (array([-0.37228132, 5.37228132]), array([[-0.82456484, -0.41597356],[ 0.56576746, -0.90937671]])) eigen value, eigen vector
# 참고: array 대신 list 로도 다음과 같이 사용할 수 있습니다.
x=[[4,2],[3,5]] # array가 아니라 list
x # [[4, 2], [3, 5]]
np.diag(x) # array([4, 5])
numpy 를 이용한 기술통계량
income = [50000, 50000, 100000, 25000, 32100, 30000]
income2 = np.array(income)
income2 # array([ 50000, 50000, 100000, 25000, 32100, 30000])
np.mean(income2) # 평균 47850.0
np.std(income2) # 표준편차 25224.574657794859
np.min(income2) # 최솟값 25000
np.max(income2) # 최댓값 100000
데이터타입 변경
d1 = np.array([1,2,3,4])
d1.dtype # dtype('int32')
d2 = np.array([1,2,3,4],dtype=np.int64)
d2.dtype # dtype('int64')
d3 = np.array([1,2,3,4], dtype=np.float32)
d3.dtype # dtype('float32')
d3 # array([ 1., 2., 3., 4.], dtype=float32)