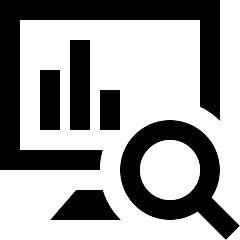
Correlations
다음 자료를 참고하였습니다:
Correlations
cor( )
함수를 사용하면 correlation 을, cov( )
함수를 사용하면 covariance 을 계산할 수 있습니다.
cor(x, use=, method= )
형태로 사용합니다.
Option | Description |
---|---|
x | Matrix or data frame |
use | Specifies the handling of missing data. Options are all.obs (assumes no missing data - missing data will produce an error), complete.obs (listwise deletion), and pairwise.complete.obs (pairwise deletion) |
method | Specifies the type of correlation. Options are pearson, spearman or kendall. |
# Correlations/covariances among numeric variables in
# data frame mtcars. Use listwise deletion of missing data.
cor(mtcars, use="complete.obs", method="kendall")
cov(mtcars, use="complete.obs")
cor( )
함수와 cov( )
함수는 significance test 를 제공하지 않습니다. cor.test( )
함수를 사용하면 single correlation coefficient test 를 할 수 있습니다.
Hmisc 패키지의 rcorr( )
함수를 사용하면 correlations/covariance, significance levels for pearson and spearman correlations 를 계산할 수 있습니다. matrix 형태로 입력해야 합니다.
# Correlations with significance levels
library(Hmisc)
rcorr(x, type="pearson") # type can be pearson or spearman
#mtcars is a data frame
rcorr(as.matrix(mtcars))
cor(X, Y)
및 rcorr(X, Y)
함수를 사용하여 X 와 Y 간의 correlation 을 계산할 수 있습니다. SAS PROC CORR
의 VAR
및 WITH
명령어와 유사합니다.
# Correlation matrix from mtcars
# with mpg, cyl, and disp as rows
# and hp, drat, and wt as columns
x <- mtcars[1:3]
y <- mtcars[4:6]
cor(x, y)
Other Types of Correlations
# polychoric correlation
# x is a contingency table of counts
library(polycor)
polychor(x)
# heterogeneous correlations in one matrix
# pearson (numeric-numeric),
# polyserial (numeric-ordinal),
# and polychoric (ordinal-ordinal)
# x is a data frame with ordered factors
# and numeric variables
library(polycor)
hetcor(x)
# partial correlations
library(ggm)
data(mydata)
pcor(c("a", "b", "x", "y", "z"), var(mydata))
# partial corr between a and b controlling for x, y, z
시각화 (Visualizing Correlations)
corrgram( )
함수를 사용하면 correlogram 을 그릴 수 있습니다.
pairs( )
혹은 splom( )
함수를 사용하면 scatterplot matrices 를 그릴 수 있습니다.
corrplot 패키지를 사용하면 heatmap 을 그릴 수 있습니다.
# 변수들 간의 상관관계 계산(numeric only)
MYDATA_corr <- cor(MYDATA)
# 변수들 간의 상관관계 히트맵 그리기
library(corrplot)
corrplot(MYDATA_corr, type="lower", diag=F, tl.col="black", tl.srt = 90)
corrplot(MYDATA_corr, type="lower", diag=F, tl.col="black", tl.srt = 90, method = "number")
# corrplot(MYDATA_corr, type="lower", diag=F, tl.col="black", tl.srt = 90, order = "hclust") # 상관성 높은 항목끼리 묶고 싶을 때
col <- colorRampPalette(c("#BB4444","#EE9988","#FFFFFF","#77AADD","#4477AA"))
corrplot(MYDATA_corr, method = "color", col=col(200), type="lower", diag=F, tl.col="black", tl.srt = 90, addCoef.col="black")