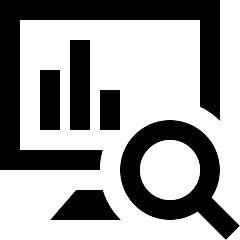
SVM (Support Vector Machine)
preface 이번 포스트에서는 분류classification 방법론 가운데 하나인 SVM (Support Vector Machine) 에 대하여 설명합니다. SVM 은 실제로 분류 정확도가 상당히 높은 방법론 가운데 하나로 알려져 있습니다.
알고리즘
다음 자료를 참고하였습니다:
- James, et. al. “An Introduction to Statistical Learning with Application in R” (이하 ISLR)
Hyperplane
SVM 을 이해하기 위해서는 우선 Maximal Margin Classifier 를 알아야 합니다. Maximal Margin Classifier 는 hyperplane 을 기준으로 분류하는 방법론입니다.
Hyperplane 이란?
in a $p$-dimensional space, a hyperplane is a flat affine1 subspace of dimension $p$-1
2차원에서 hyperplane 은 다음과 같은 식으로 정의됩니다.
(ISLR eq 9.1):
동일하게, $p$-차원에서 hyperplane 은 다음과 같은 식으로 정의됩니다.
(ISLR eq 9.2):
$p$-차원에 있는 점 $X = \left( X_1, X_2, … , X_p \right)^T$ 는 위 hyperplane 을 기준으로 그 위치를 나눌 수 있습니다.
(ISLR eq 9.3):
(ISLR eq 9.4):
$i$ 번째 관측치 $y_i$ 가 $y_i \in \left( -1, 1 \right)$ 와 같이 두 가지 class 로 분류될 때, 이들을 분류하는 separating hyperplane 은 다음과 같은 성질을 가지고 있습니다.
(ISLR eq 9.6):
(ISLR eq 9.7):
위 두 식을 하나로 나타내면, separating hyperplane 은 다음과 같은 성질을 지닌다고 할 수 있습니다.
(ISLR eq 9.8):
Maximal Margin Classifier
$p$-차원에서 $X$ 가 보기에도 확연하게 두 그룹으로 나뉘어있다면, 그룹을 나누는 separating hyperplane 을 여러개 만들 수 있습니다.
Maximal Margin Classifier 는 이러한 separating hyperplane 가운데 양 그룹의 관측치로부터 가장 멀리 떨어진 maximal margin hyperplane 을 선택하는 방법입니다.
maximal margin hyperplane is the separating hyperplane that is farthest from the training observations
이때, maximal margin hyperplane 으로부터 가장 가까운 관측치, 즉 maximal margin hyperplane 을 정의하는 관측치를 supprot vector 라고 합니다.
[ISLR Figure 9.3 참조]
maximal margin hyperplane 은 다음 optimization problem 을 통해서 구할 수 있습니다.
(ISLR eq 9.9):
Support Vector Classifier
maximal margin hyperplane 은 관측치가 확연히 구분되지 않는 경우, 즉 양 그룹이 섞여있는 경우 이를 구할 수 없습니다.
경우에 따라서는 separating hyperplane 이 양 그룹을 완벽하게 구분짓지 않더라도, 즉 약간의 errors 를 허용하는 것이 더 좋은 구분 방법이 될 수도 있습니다. 여기에서 등장한 개념이 support vector classifier, 혹은 soft margin classifier 입니다.
supprot vector classifier 는 다음 optimization problem 을 통해서 구할 수 있습니다.
(ISLR eq 9.12):
where $C$ is a nonnegative tuning parameter
약간의 error 를 허용하되, 그 총합이 $C$ 를 넘지 않도록 하겠다는 것입니다. 실제 분석에서 $C$ 는 일반적으로 cross-validation 을 통해 선택됩니다.
Support Vector Machines
지금까지 support vector classifier 는 $X$ 의 linear combination 으로 정의되었습니다. 이번에는 여기에 non-linearity 를 추가하고자 합니다.
지금까지 $X_1, X_2, …, X_p$ 라는 $p$ 개의 features 를 사용한 support vector classifier 대신, 이번에는 $X_1, X_1^2, X_2, X_2^2, …, X_p, X_p^2$ 라는 $2p$ 개의 features 를 사용합니다. 이렇게 정의된 classifier 는 다음과 같이 표현할 수 있습니다.
(ISLR eq 9.16):
Support Vector Machine (SVM) 은 이러한 support vector classifier 를 kernel 함수를 이용하여 feature space 를 확장한 것입니다.
벡터 간의 내적(inner product) 기호를 사용하면 linear support vector classifier 를 보다 간단하게 표시할 수 있습니다.
inner product 는 다음과 같이 표현합니다.
(ISLR eq 9.17):
inner product 기호를 사용하면 linear support vector classifier 를 다음과 같이 표현할 수 있습니다.
(ISLR eq 9.18):
이때 $x_i$ 가 support vector 가 아닌 경우 $\alpha_i = 0$ 이 되므로, support vector 의 집합을 $S$ 라 표현한다면 다음과 같이 나타낼 수 있습니다.
(ISLR eq 9.19):
이러한 inner product 를 보다 일반화하여, kernel 함수 $K$ 로 표현한다면 다음과 같이 쓸 수 있습니다.
(ISLR eq 9.23):
많이 사용되는 kernel 함수로는 linear, ploynomial, raidal kernel 이 있습니다.
(ISLR eq 9.21) linear kernel:
(ISLR eq 9.22) polynomial kernel:
(ISLR eq 9.24) radial kernel:
SVM in Python by using scikit-learn
Hyperplane
두 그룹으로 나뉘는 샘플을 랜덤하게 생성하고, 이를 hyperplane 으로 나누는 예제입니다.
import numpy as np
import matplotlib.pyplot as plt
from sklearn import svm
%matplotlib inline
# we create 40 separable points
np.random.seed(0)
X = np.r_[np.random.randn(20, 2) - [2, 2], np.random.randn(20, 2) + [2, 2]]
Y = [0] * 20 + [1] * 20
# fit the model
clf = svm.SVC(kernel='linear')
clf.fit(X, Y)
# get the separating hyperplane
w = clf.coef_[0]
a = -w[0] / w[1]
xx = np.linspace(-5, 5)
yy = a * xx - (clf.intercept_[0]) / w[1]
# yy = (-w[0] / w[1]) * xx - (clf.intercept_[0]) / w[1]
# w[1]*yy = -w[0]*xx - clf.intercept_[0]
# clf.intercept_[0] + w[0]*xx + w[1]*yy = 0
# equivalent to \beta_0 + \beta_1 * x_1 + \beta_2 * x_2 = 0
# plot the parallels to the separating hyperplane that pass through the
# support vectors
b = clf.support_vectors_[0]
yy_down = a * xx + (b[1] - a * b[0])
b = clf.support_vectors_[-1]
yy_up = a * xx + (b[1] - a * b[0])
# plot the line, the points, and the nearest vectors to the plane
plt.plot(xx, yy, 'k-')
plt.plot(xx, yy_down, 'k--')
plt.plot(xx, yy_up, 'k--')
plt.scatter(clf.support_vectors_[:, 0], clf.support_vectors_[:, 1],
s=80, facecolors='none')
plt.scatter(X[:, 0], X[:, 1], c=Y, cmap=plt.cm.Paired)
plt.axis('tight')
SVM
Iris 데이터를 사용합니다. 그래프로 표현하기 위하여 4가지 features 중 2가지 features 만 사용하였습니다. 예측 정확도 테스트를 위하여 train set 과 test set 으로 나누었습니다.
import numpy as np
import matplotlib.pyplot as plt
from sklearn import svm, datasets
from sklearn.model_selection import train_test_split
iris = datasets.load_iris()
X = iris.data[:, :2]
y = iris.target
X_train, X_test, y_train, y_test = train_test_split(X, y, test_size=0.33, random_state=42)
SVM 을 실행합니다. kernel 함수를 지정할 수 있습니다.
# %matplotlib inline # jupyter notebook 인 경우 uncomment 합니다.
h = .02
C = 1.0
svc = svm.SVC(kernel='linear', C=C).fit(X_train, y_train)
rbf_svc = svm.SVC(kernel='rbf', gamma=0.7, C=C).fit(X_train, y_train)
poly_svc = svm.SVC(kernel='poly', degree=3, C=C).fit(X_train, y_train)
lin_svc = svm.LinearSVC(C=C).fit(X_train, y_train)
데이터와 함께 예측된 구역을 나눕니다.
x_min, x_max = X[:, 0].min() - 1, X[:, 0].max() + 1
y_min, y_max = X[:, 1].min() - 1, X[:, 1].max() + 1
xx, yy = np.meshgrid(np.arange(x_min, x_max, h),
np.arange(y_min, y_max, h))
# title for the plots
titles = ['SVC with linear kernel',
'LinearSVC (linear kernel)',
'SVC with RBF kernel',
'SVC with polynomial (degree 3) kernel']
for i, clf in enumerate((svc, lin_svc, rbf_svc, poly_svc)):
plt.subplot(2, 2, i + 1)
plt.subplots_adjust(wspace=0.4, hspace=0.4)
Z = clf.predict(np.c_[xx.ravel(), yy.ravel()])
# Put the result into a color plot
Z = Z.reshape(xx.shape)
plt.contourf(xx, yy, Z, cmap=plt.cm.coolwarm, alpha=0.8)
# Plot also the training points
plt.scatter(X_train[:, 0], X_train[:, 1], c=y_train, cmap=plt.cm.coolwarm)
plt.xlabel('Sepal length')
plt.ylabel('Sepal width')
plt.xlim(xx.min(), xx.max())
plt.ylim(yy.min(), yy.max())
plt.xticks(())
plt.yticks(())
plt.title(titles[i])
X_test 를 사용하여 예측력을 테스트합니다.
clf.predict(X_test)
clf.score(X_test, y_test)
71.99 % 의 정확도를 나타냅니다.
-
The word affine indicates that the subspace need not pass through the origin. ↩